Informatics Institute faculty member Behçet Uğur Töreyin and Abdulkerim Çapar coauthored paper titled 'Automated scoring of CerbB2/HER2 receptors using histogram based analysis of immunohistochemistry breast cancer tissue images' has been published in 'Biomedical Signal Processing and Control' in 2021.
DOI: https://doi.org/10.1016/j.bspc.2021.102924
Cite:
Kabakçı, K.A., Çakır, A., Türkmen, İ., Töreyin, B.U. and Çapar, A., 2021. Automated scoring of CerbB2/HER2 receptors using histogram based analysis of immunohistochemistry breast cancer tissue images. Biomedical Signal Processing and Control, 69, p.102924.
Abstract:
Background and Objective: Visual expression of invasive breast cancer with immunohistochemistry (IHC) allows evaluation of CerbB2 receptors, such that CerbB2 mutated breast carcinomas are suitable for targeted therapy. Breast tumors are evaluated in four different scores as 0, 1, 2, 3 to decide if it is suitable for the CerbB2 protein specific treatment or not. Pathologists try to decide the scores by eye, which is laborious, and error-prone work with high inter-observer variability.
Methods:
We propose cell based image analysis termine the CerbB2/HER2 scores in breast tissue images in accordance with ASCO/CAP recommendations, automatically. The proposed ASCO/CAP recommendations compliant image analysis approach provides an explainable artificial intelligence solution for HER2 tissue scoring. Firstly, tissue images are separated into hematoxylin and diaminobenzidine color channels with color deconvolution. Cell nuclei and boundaries are segmented with a hybrid multi-level thresholding and radial line based method on hematoxylin channel. Following ASCO/CAP recommendations, cell based features representing the intensity and completeness of circumferential membrane staining are extracted with the proposed Membrane Intensity Histogram (MIH) method. Extracted features are, then, fed into a classifier, such as, k-nearest neighbours, decision trees and long-short term memory, to determine cell based HER2 scores. Individual cell scores are combined according to ASCO/CAP recommendations to obtain the final CerbB2/HER2 tissue score. Another contribution of the paper is the introduction of two publicly available image data sets on CerbB2/HER2 tissue scoring. Clinical data sets, ITU-MED-1 and ITU-MED-2, are created by digitizing IHC slides from real patients, that have ground truth CerbB2/HER2 scores.
Result:
The proposed automatic scoring method is tested on these clinical data sets, as well as, on a HER2 Contest data set. Performance of the proposed explainable artificial intelligence approach for HER2 tissue scoring is evaluated and compared with state-of-the-art techniques in the literature.
Conclusion:
Results suggest that, the proposed method is highly effective in HER2 tissue scoring on both balanced and unbalanced data sets.
Significance:
A hand-crafted feature extraction approach for CerbB2/HER2 scoring is proposed which provides an explainable artificial intelligence framework. The proposed HER2 scoring method can be adapted to updates in ASCO/CAP recommendations without the need for re-training and/or re-designing the model. Moreover, two publicly available data sets, namely, ITU-MED-1 and ITU-MED-2 are introduced with corresponding score labels.
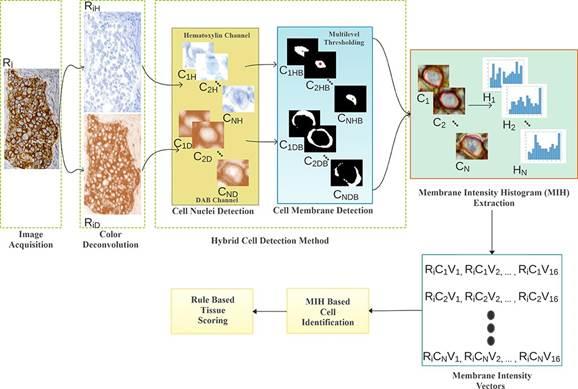